On generalized cloud infrastructures, most businesses are forced to make a decision between using a low-powered, general-purpose GPU that fits their budget, or finding the performance they need from a high-end GPU with higher costs. This can limit certain use cases, like model serving, for companies looking to build products and applications using the latest developments in AI.
A major benefit of working with a specialized cloud provider is the ability to choose from a wide selection of GPUs to ensure that you are able to match the complexity of your workloads with compute that delivers an effective and scalable performance-adjusted cost. With the broadest selection of GPUs on the market, CoreWeave practically eliminates the need to choose between price or performance, empowering our clients to get the performance they need with economics that empower scale.
We encourage all of our clients to benchmark their workloads to find what works best for them. Coupled with the industry’s fastest spin-up times and most responsive auto-scaling delivered out-of-the-box with CoreWeave’s InferenceService, finding the best GPUs for the models you’re serving can reduce the amount of idle compute you consume, serve end-user demand faster in real-time, and lower inference latency while only paying for what you use.
To help you get started, here’s some guidance on how we think about our arsenal of NVIDIA GPUs for model serving:
NVIDIA Quadro RTX 4000
Just because the NVIDIA Turing architecture-based NVIDIA Quadro RTX™ 4000 is the smallest GPU that CoreWeave offers doesn't mean it's not cost-effective. If you need to run inference for models such as the Fairseq 2.7B or GPT Neo 2.7B or smaller, this can be an excellent value for less intensive inference workloads.
Larger contexts may require the Quadro RTX 5000 described below, depending on how efficient your inference engine is. However, if you are saturating the GPU with inference requests, then the more recent GPUs such as the NVIDIA RTX A4000 or A5000 may serve you better - read on for more on those options.

NVIDIA RTX 5000
The Turing-based NVIDIA® Quadro RTX 5000 is the smallest GPU that can run inference for the GPT-J 6B or Fairseq 6.7B models. It has double the RAM, a bit more memory bandwidth than the RTX 4000 and a much faster base clock rate.
If your 2.7B models are running out of RAM with a larger context, this is the next step up and will give you faster inference to boot.
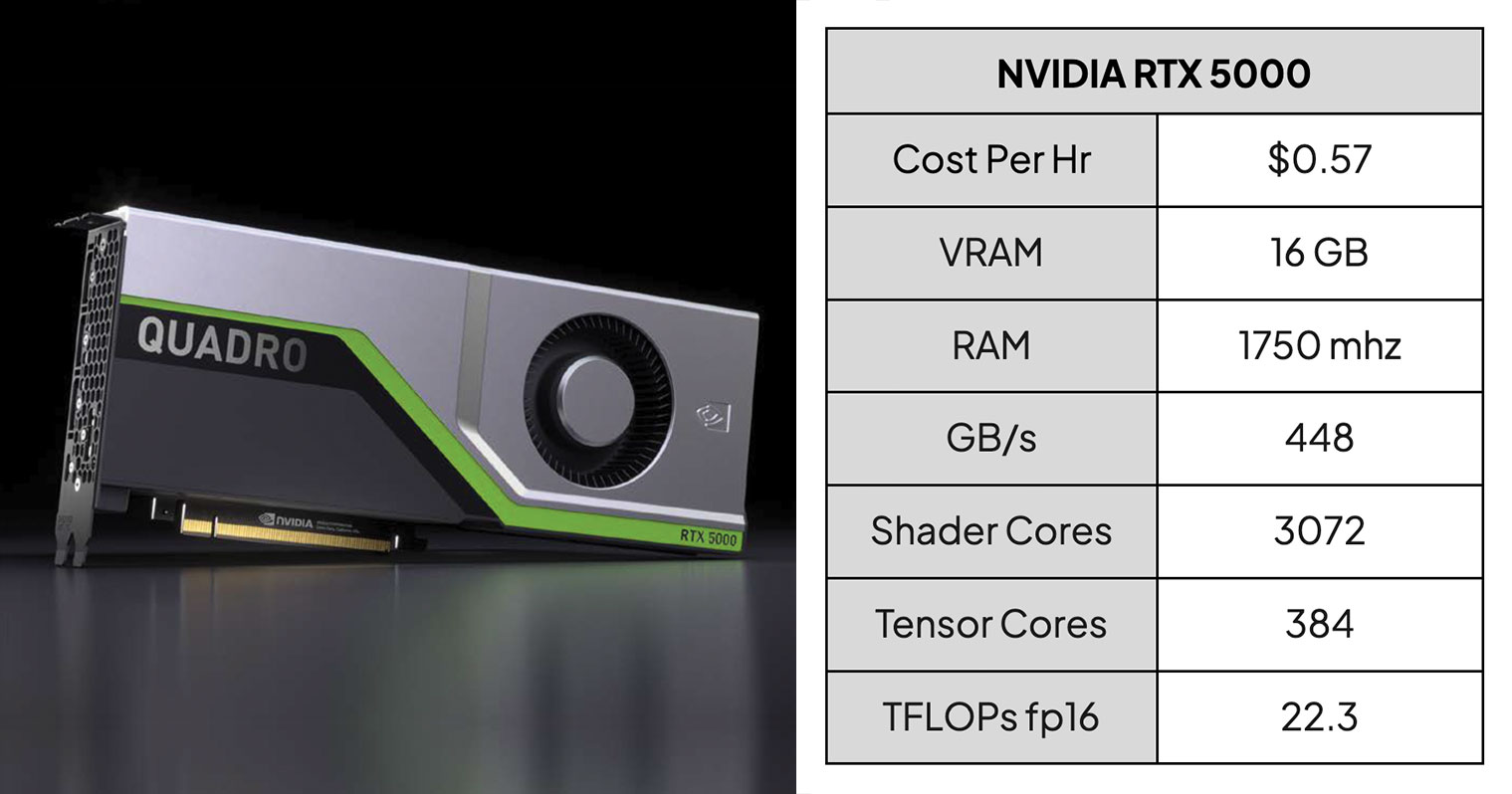
NVIDIA Quadro RTX A4000
The NVIDIA Ampere architecture-based NVIDIA RTX A4000 is a small step up from the Quadro RTX 5000, although it may not look like it at first glance. The clock rate is half that of the Quadro RTX 5000, but the boost clock nearly matches the base clock of the older GPU. What makes the difference is the number of shader cores, which is doubled.
However, the number of tensor cores is half that of the Quadro RTX 5000. Whether the RTX A4000 or the Quadro RTX 5000 work better for your workload depends on your inference framework and what instructions you use.
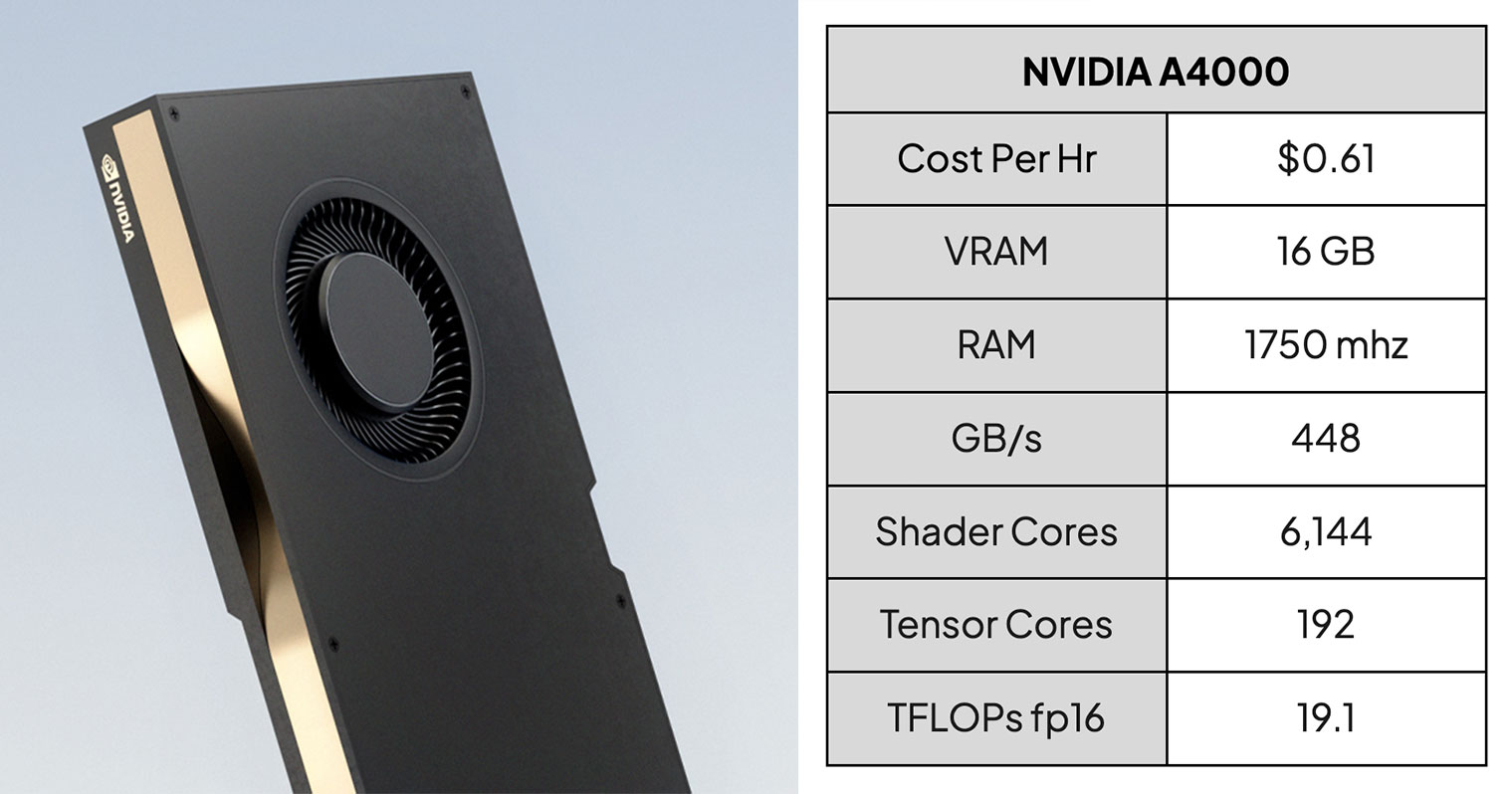
NVIDIA RTX A5000
The NVIDIA Ampere architecture-based NVIDIA RTX A5000 is a good step up from the RTX A4000 and has been observed to be faster at running GPT-J 6B and Fairseq 6.7B than the A4000 for inference. It is also the smallest NVIDIA GPU that can be comfortably used for fine-tuning smaller models, such as Fairseq, GPT Neo 1.3B or GPT Neo 2.7B.
If your model fits comfortably inside 24GB, this GPU is a better value proposition than the RTX A6000. It can also host the Fairseq 13B model for inference, although it is tight at 24GB.
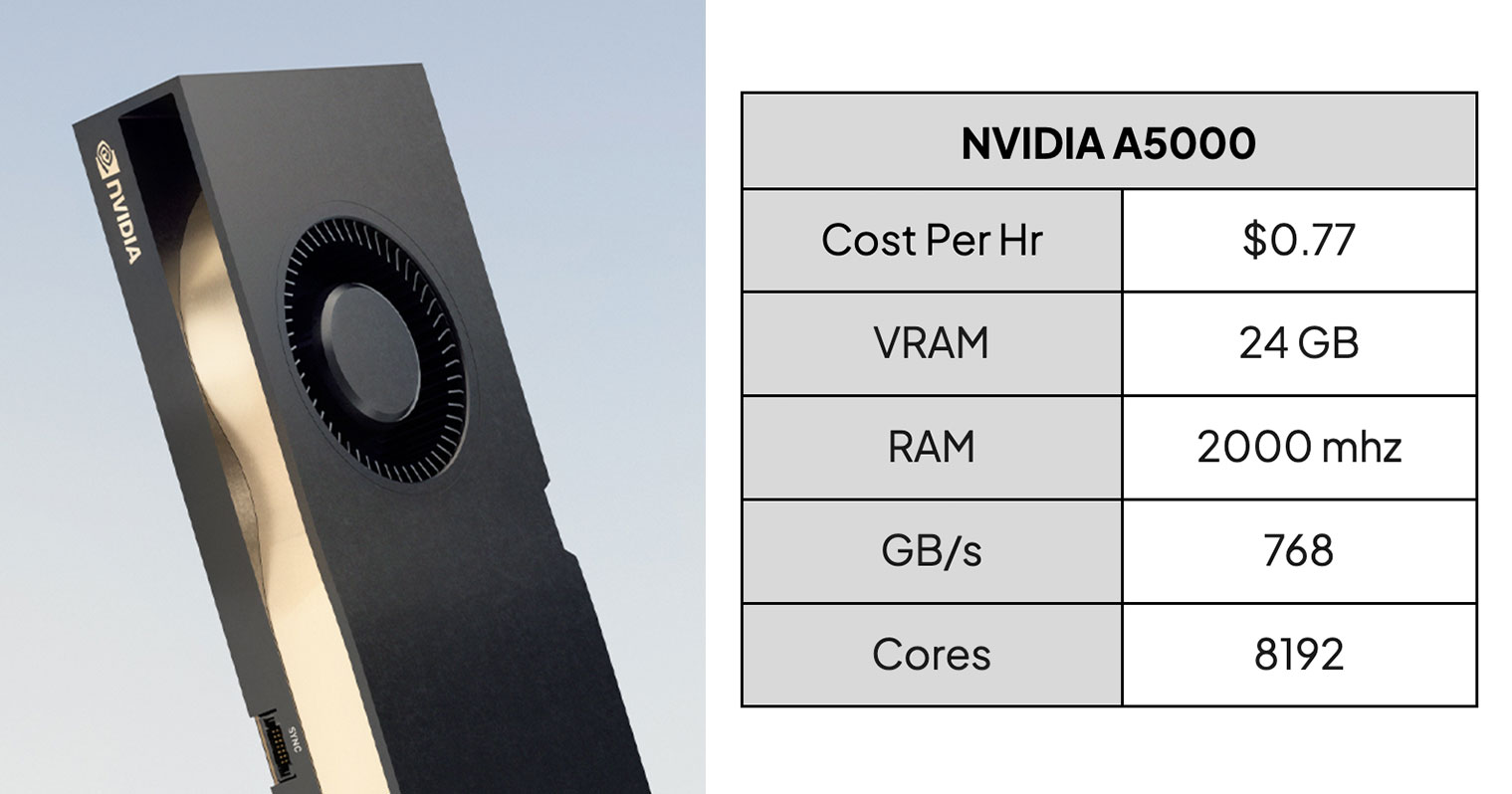
NVIDIA RTX A6000
If your workload is intense enough, the NVIDIA Ampere architecture-based NVIDIA RTX A6000 is one of the best values for inference. It is CoreWeave's recommended GPU for fine-tuning, due to the 48GB of RAM, which allows you to fine-tune up to Fairseq 13B on a single GPU. The 48GB of RAM also allows you to batch-train steps during fine-tuning for better throughput.
The RTX A6000 is the smallest single NVIDIA GPU that can host the GPT NeoX 20B model.
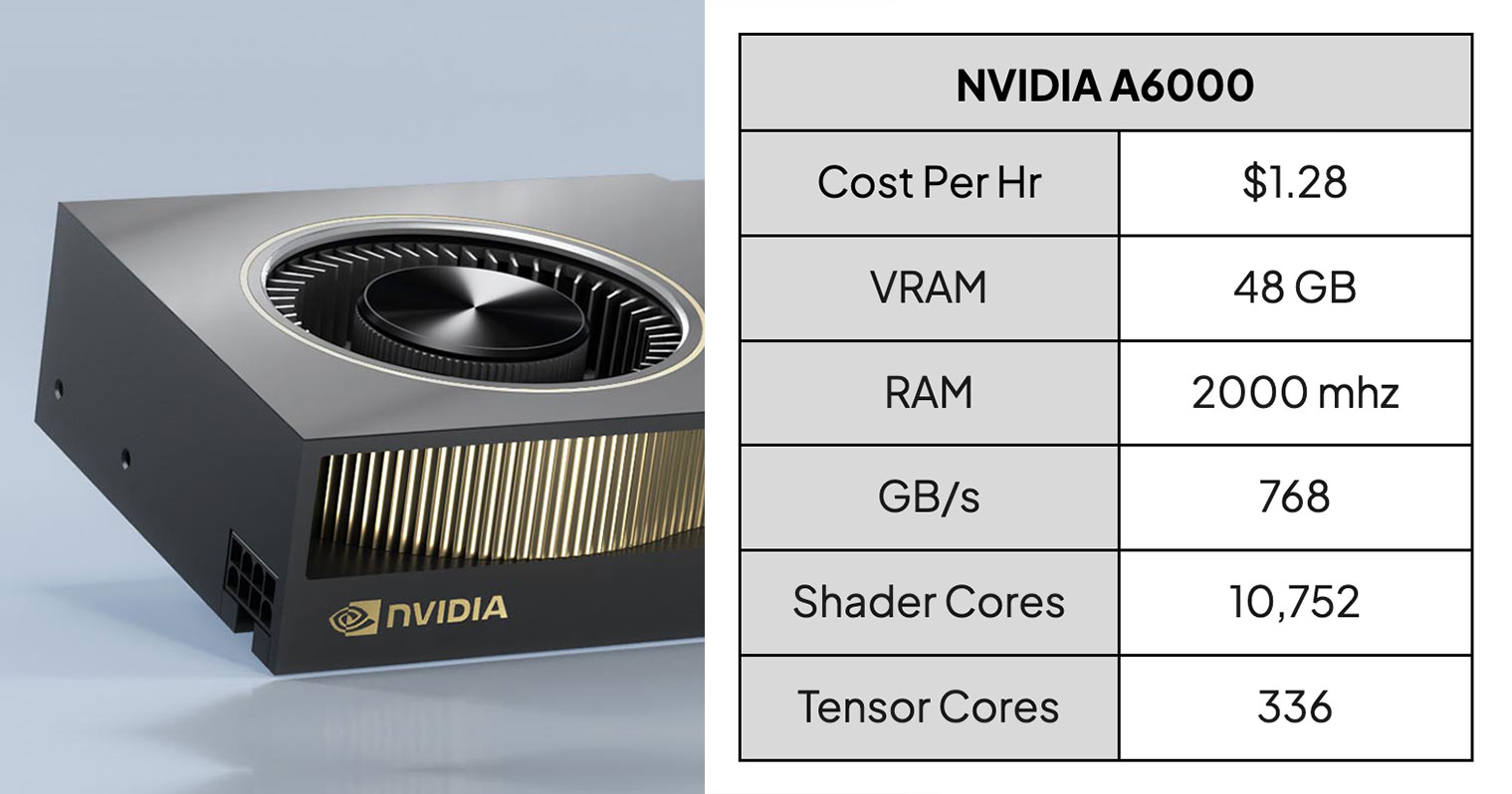
NVIDIA A40
Because of the value proposition, the NVIDIA A40 is our recommended GPU for larger-scale training jobs. The RTX A6000 is slightly faster, but the A40 has more robust GPU drivers and more availability at CoreWeave. CoreWeave can help with setting this up.
The A40’s 48GB of RAM allows you to batch-train steps during fine-tuning for better throughput and the CoreWeave Finetuning Machine Learning Models Guide defaults to the A40 for this reason.
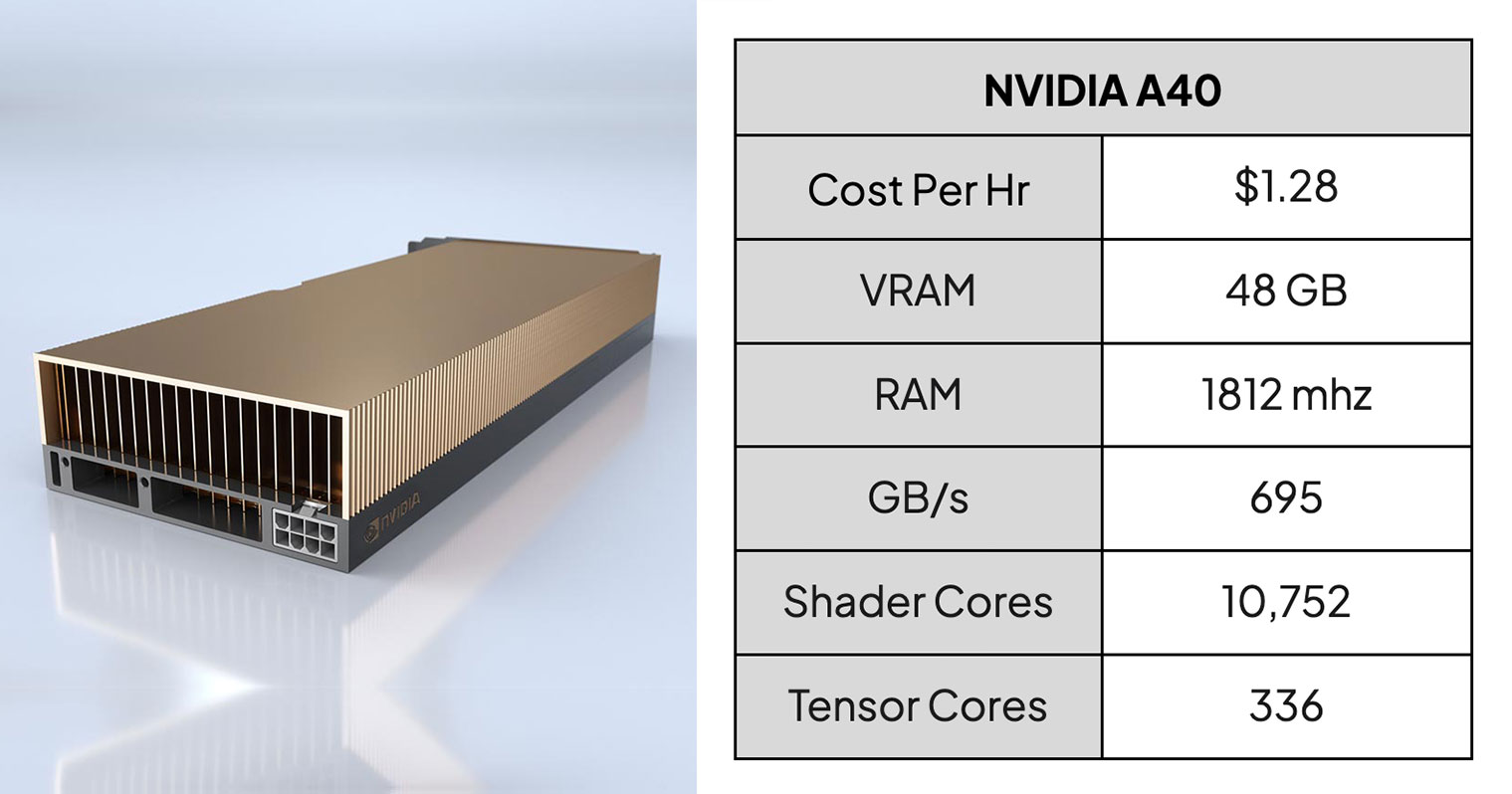
NVIDIA A100 40GB
The NVIDIA A100 40GB PCIe GPU nearly doubles the performance of the NVIDIA A40/RTX A6000 on a single GPU basis for many workloads due to double the memory bandwidth. However, it has 8GB less RAM than the A40, which makes the latter better suited to host larger models such as GPT NeoX 20B on a single GPU.
Pairs of NVIDIA A100 PCIe GPUs can make excellent inference nodes if inference throughput is your primary concern. The NVIDIA NVLink interconnect is recommended for distributed training and inference when model parallelism is required.
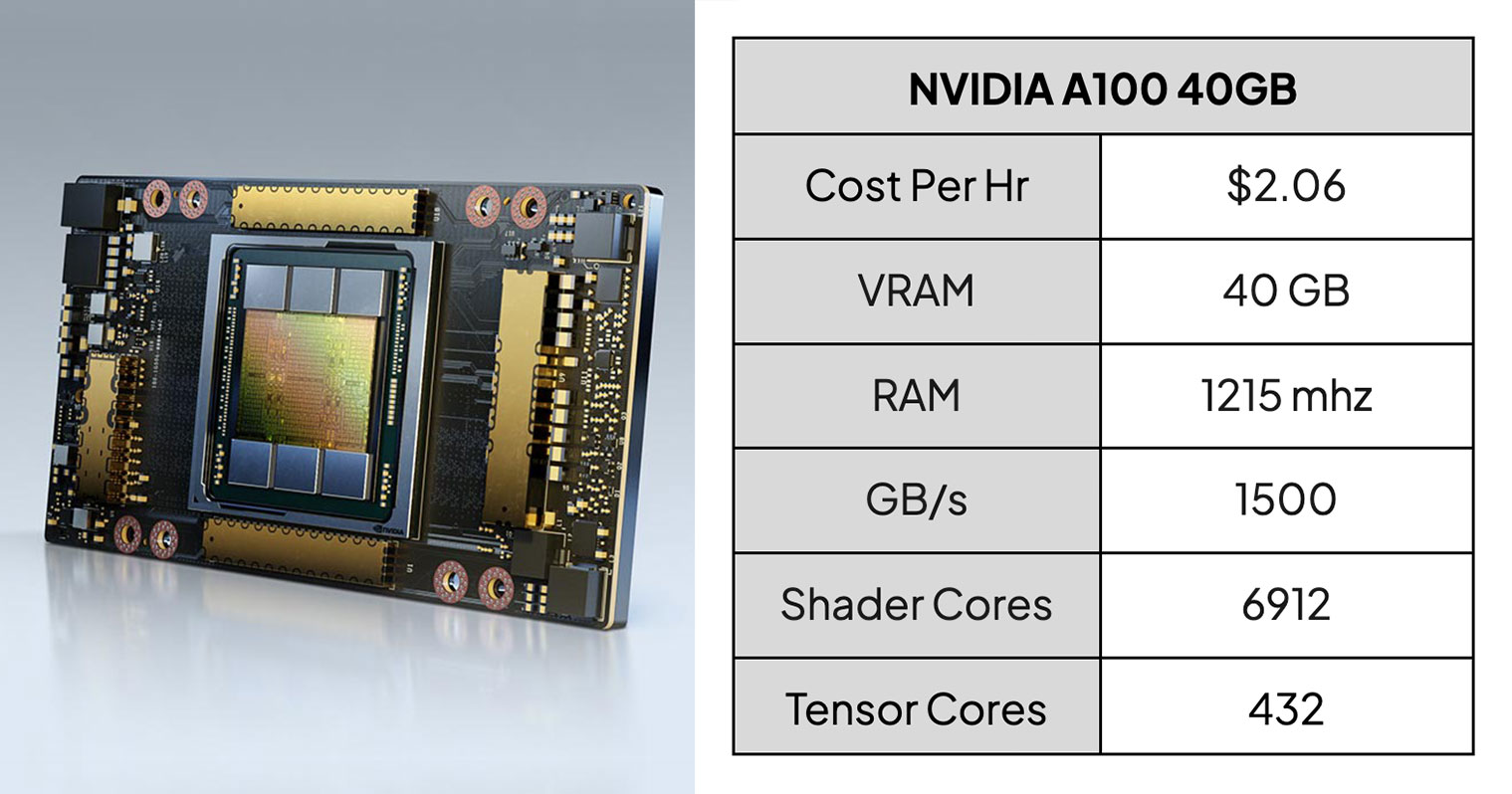
NVIDIA A100 80GB
With double the RAM and 30% more memory bandwidth than the NVIDIA A100 40GB PCIe GPU, this is our top choice for large model inference on CoreWeave with a single GPU. 20B parameter models run as fast and comfortably on an NVIDIA A100 80GB PCIe as 13B parameter models do on an RTX A6000.
The NVIDIA NVLink interconnect is recommended for distributed training and inference when model parallelism is required.
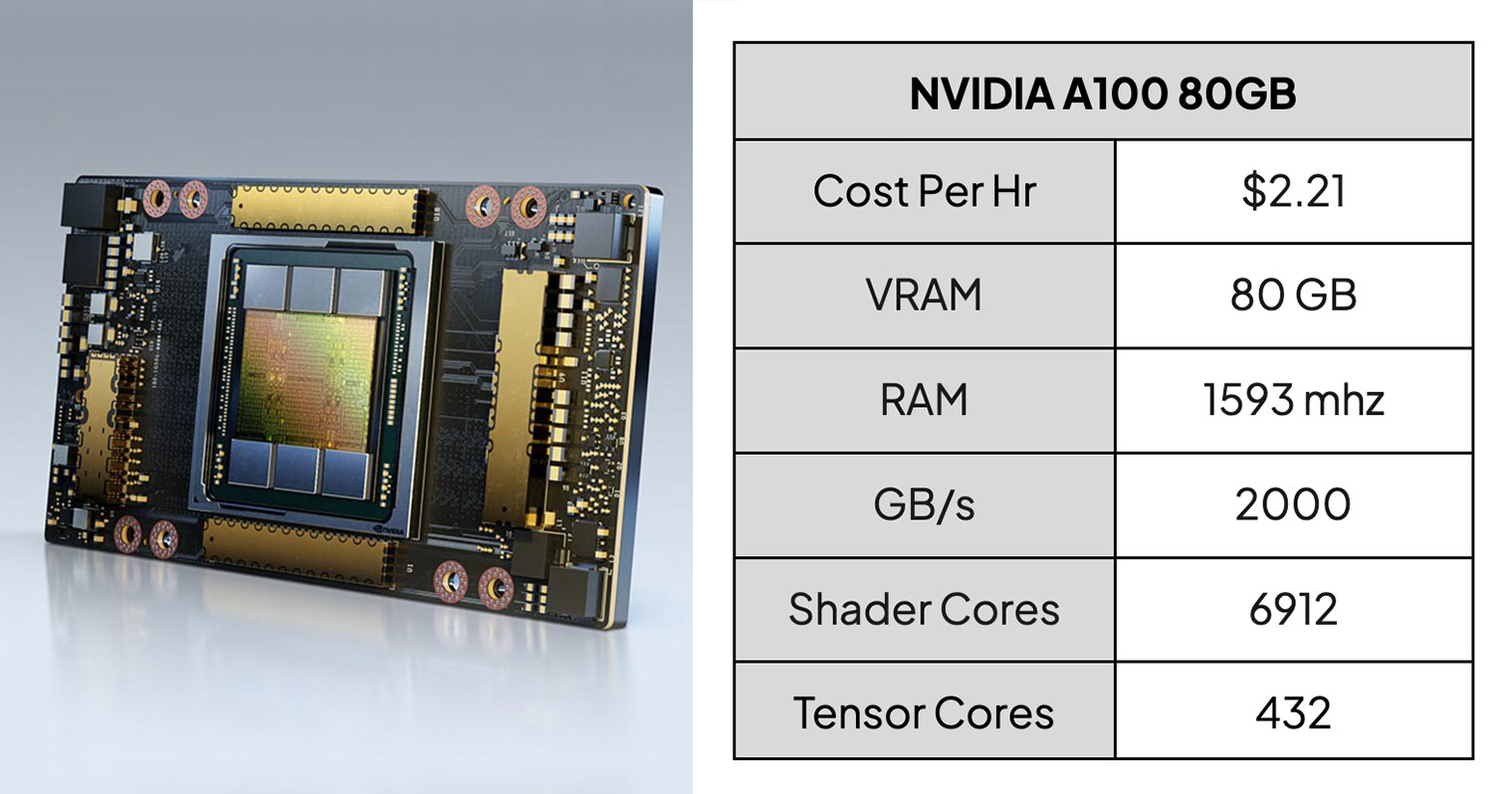
The CoreWeave support team is always ready to roll up our sleeves and help guide our clients through benchmarking workloads, maximizing our InferenceService and getting the absolute most out of the entire CoreWeave tech stack. We have a rich library of inference examples, including documentation on serving BLOOM 176B, GPT-J-6B and more.
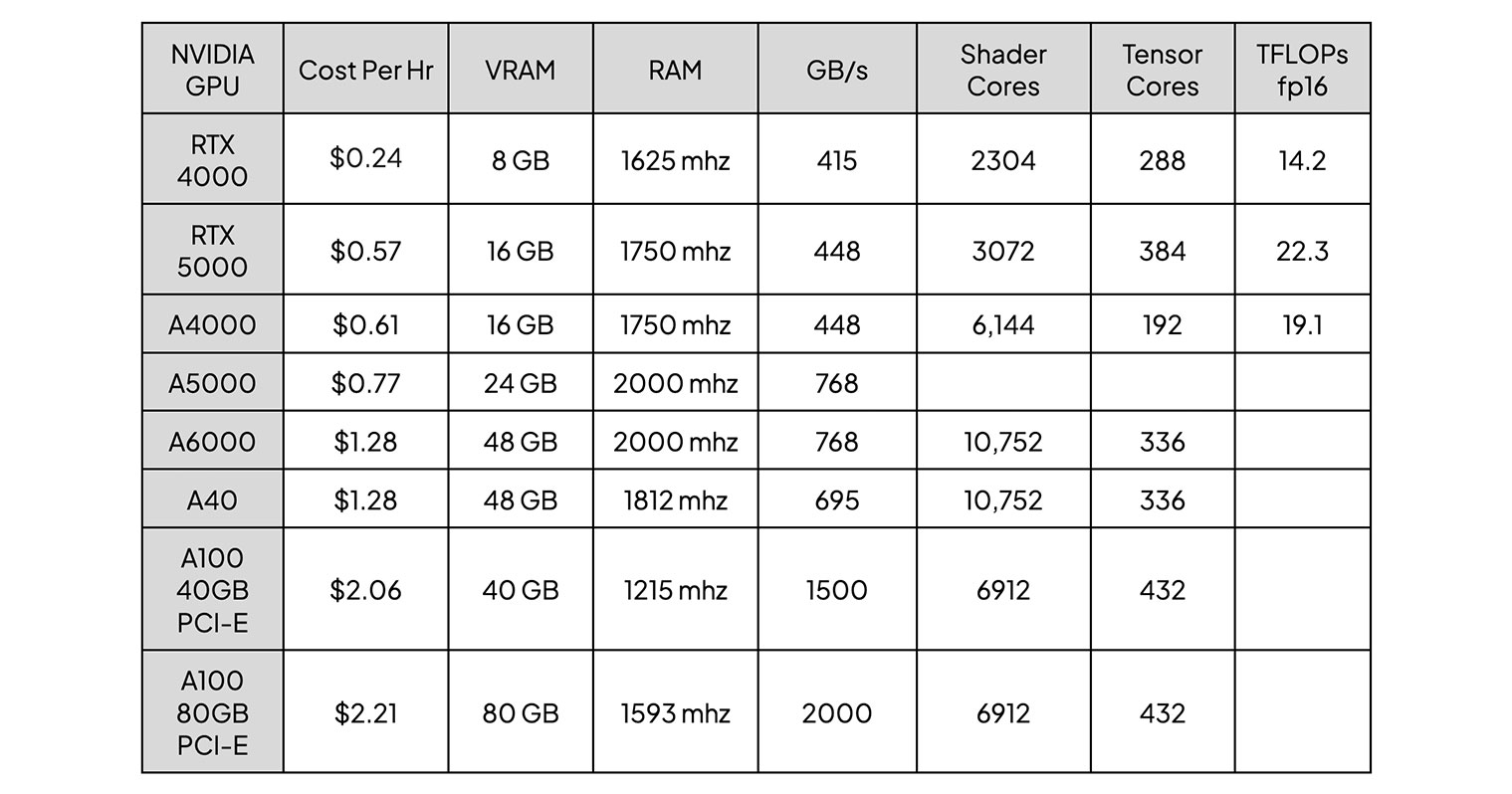
Get In Touch
CoreWeave is partnering with companies around the world to fuel AI & natural language processing development, and we’d love to help you too! Get started by speaking with one of our engineers today.